19/04/2025
19/04/2025
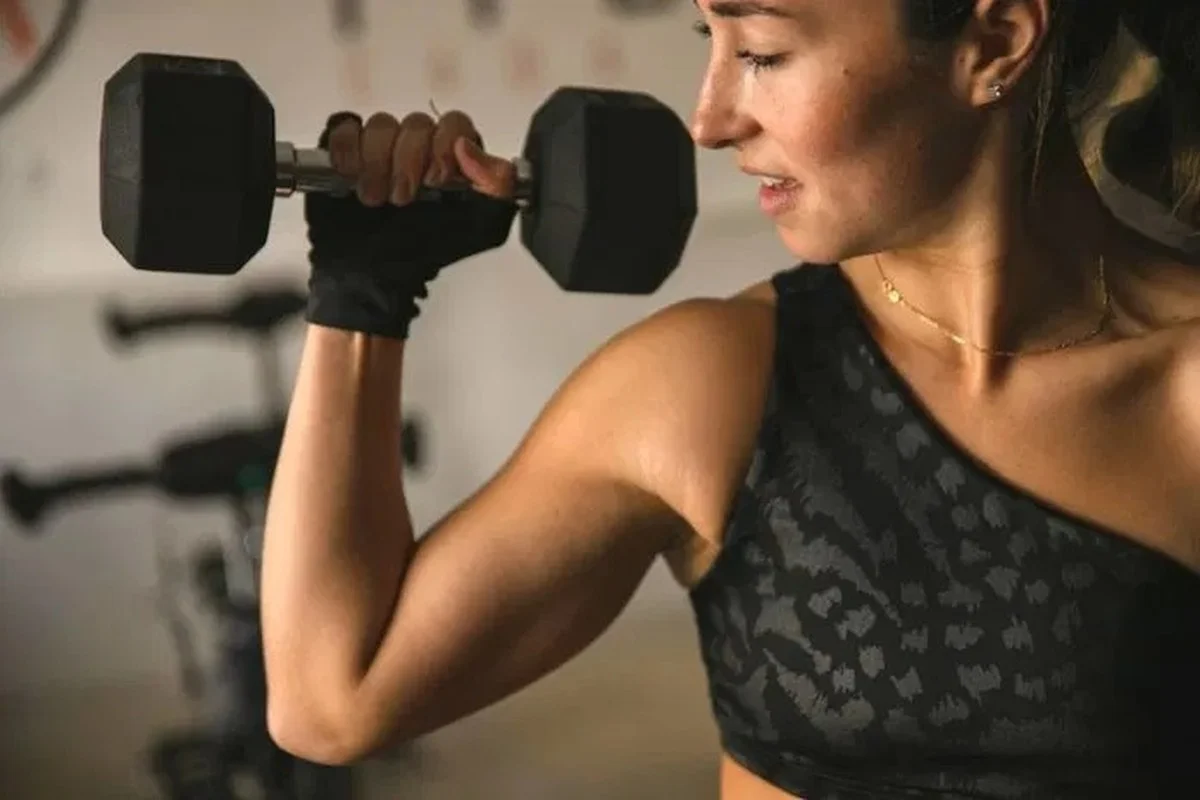
NEW YORK, April 19: A team of researchers at the University of Mississippi has harnessed the power of machine learning to identify the strongest predictors of long-term exercise habits. Analyzing data from nearly 12,000 individuals, the study reveals that sedentary time, gender, and education level are the top indicators of whether someone consistently meets recommended physical activity guidelines.
The findings, published in the journal Scientific Reports, could reshape how public health officials and fitness professionals tailor strategies to promote regular physical activity.
Led by doctoral students Seungbak Lee and Ju-Pil Choe, and professor Minsoo Kang from the Department of Health, Exercise Science and Recreation Management, the research team trained machine learning models on data drawn from the National Health and Nutrition Examination Survey (NHANES), spanning 2009 to 2018. The survey includes detailed lifestyle, demographic, and health-related responses.
“We wanted to use advanced data analytic techniques, like machine learning, to predict this behavior,” Kang explained. “Physical activity adherence to the guidelines is a public health concern because of its relationship to disease prevention and overall health patterns.”
The U.S. Department of Health and Human Services recommends adults engage in at least 150 minutes of moderate exercise or 75 minutes of vigorous activity weekly. Yet, the average American spends only about two hours per week on physical activity—far short of the four-hour weekly benchmark set by the CDC.
The researchers explored a wide range of factors including age, gender, race, income, education, marital status, BMI, waist circumference, sleep, alcohol use, and smoking habits. Participants with certain diseases or missing data on physical activity were excluded, resulting in a final sample size of 11,683.
While several variables were considered, the machine learning models consistently identified three as most predictive: time spent sitting, gender, and education level. These factors appeared across all high-performing predictive models, suggesting a strong link to exercise commitment.
“I expected that factors like gender, BMI, race or age would be important,” said Choe, the study’s lead author. “But I was surprised by how significant educational status was. While gender or BMI are innate, education is an external factor that might influence behavior over time.”
The study’s reliance on self-reported activity levels is one of its limitations, as participants often overestimate their physical activity. The researchers note that future studies could be strengthened by incorporating wearable fitness data for more objective results.
Nevertheless, the team believes that machine learning offers a powerful approach to understanding health behavior patterns. Unlike traditional statistical methods that assume linear relationships, machine learning can identify more nuanced connections between variables.
Looking ahead, the researchers plan to use similar methods to explore other health behaviors, such as diet and supplement use, with the goal of helping fitness experts and health policymakers develop personalized, sustainable workout strategies.
“Ultimately, we want to support programs that help people stay active long-term,” said Kang. “Understanding what keeps them going is the first step.”